El futuro de la experimentación con haces de partículas: un nuevo algoritmo innovador mejora nuestra comprensión
2 min read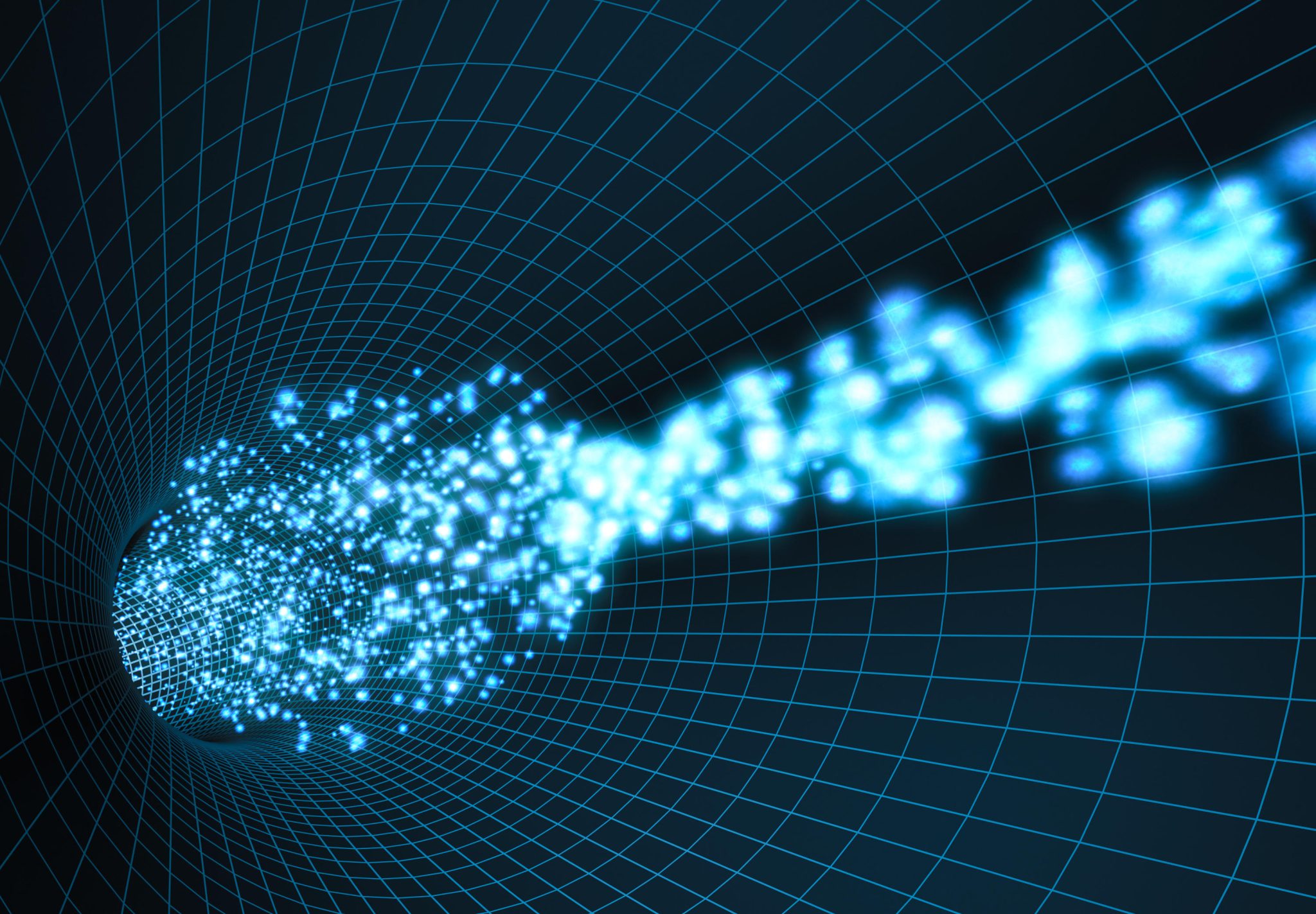
Representación gráfica de un haz de partículas en un acelerador. Crédito: Greg Steward/Laboratorio Nacional de Aceleradores de SLAC
El algoritmo combina ecuaciones clásicas de física de haces con técnicas de aprendizaje automático para reducir la necesidad de un extenso procesamiento de datos.
Cuando el acelerador lineal del SLAC National Accelerator Laboratory está funcionando, grupos de alrededor de mil millones de electrones se mueven a través de tuberías de metal casi a la velocidad de la luz. Estos grupos de electrones forman el haz de partículas del acelerador, que se utiliza para estudiar el comportamiento atómico de las moléculas, los materiales innovadores y muchos otros temas.
Sin embargo, determinar cómo se ve realmente un haz de partículas cuando viaja a través de un acelerador es un desafío, lo que deja a los científicos solo con una estimación aproximada de cómo se comportará el haz durante un experimento.
Ahora, investigadores de SLAC en el Departamento de Energía, el Laboratorio Nacional Argonne del DOE y la Universidad de Chicago han desarrollado un algoritmo que predice con mayor precisión la distribución de posiciones y velocidades de partículas en un haz cuando pasa a través de un acelerador.
Esta información detallada del haz ayudará a los científicos a realizar sus experimentos de manera más confiable, una necesidad que se está volviendo cada vez más importante a medida que las instalaciones de aceleradores operan a energías cada vez más altas y generan perfiles de haz más complejos. Los investigadores detallaron su algoritmo y método en abril de 2023 en la revista
“We have a lot of different ways to manipulate particle beams inside of accelerators, but we don’t have a really precise way to describe a beam’s shape and momentum,” SLAC accelerator scientist and lead co-author Ryan Roussel said. “Our algorithm takes into account information about a beam that is normally discarded and uses that information to paint a more detailed picture of the beam.”
Typically, researchers describe the positions and speeds of particles in a beam in terms of a few summary statistics that provide a rough shape of the beam overall – but that approach throws out a lot of potentially useful information. Alternatively, beam scientists can take many measurements of the beam itself and try to reconstruct, sometimes using machine learning, what the beam would look like under different experimental circumstances – but those methods require a lot of data and a lot of computational power.
For this study, the team tried a new approach: They built a machine-learning model that uses our understanding of beam dynamics to predict the distribution of particles positions and speeds within the beam, collectively known as the beam’s phase space distribution.
To test their ideas, the team used their model to interpret experimental data from the Argonne Wakefield Accelerator at the DOE’s Argonne National Laboratory. Including the physics of particle beam dynamics with the experimental data allowed the researchers to accurately reconstruct fine details of the beam using only 10 data points – a task that might take up to 10,000 data points for some machine learning models that don’t include a model of beam physics.
“Most machine learning models don’t directly include any notion of particle beam dynamics to speed up learning and reduce the amount of data required,” SLAC accelerator scientist and co-author Auralee Edelen said. “We’ve shown that we can infer very complicated high-dimensional beam shapes from astonishingly small amounts of data.”
The algorithm can currently reconstruct a model of a beam along its up-down and left-right axes, as if the particle bunch were a pancake moving down the accelerator path. This type of reconstruction is called 4D beam phase space. Next, researchers want to demonstrate the algorithm experimentally on reconstructing full 6D phase space distributions, which include particle positions and speed along the direction in which the beam is traveling.
Overall, the algorithm is a major paradigm shift in the way we analyze experimental accelerator data at facilities today, Roussel said.
“We can now use particle beam data in a more comprehensive, powerful way to improve our scientific goals at accelerators everywhere,” he said.
Reference: “Phase Space Reconstruction from Accelerator Beam Measurements Using Neural Networks and Differentiable Simulations” by R. Roussel, A. Edelen, C. Mayes, D. Ratner, J. P. Gonzalez-Aguilera, S. Kim, E. Wisniewski and J. Power, 5 April 2023, Physical Review Letters.
DOI: 10.1103/PhysRevLett.130.145001
“Increíble aficionado a la música. Estudiante. Empollón empedernido del café. Jugador. Especialista web aficionado. Pionero malvado de la cultura pop”.